Research
Other Projects
Emotional Speech Conversion
Emotion is the cornerstone of human interactions. In fact, the manner in which something is said can convey just as much information as the words being spoken. Emotional cues in speech are conveyed through vocal inflections known as prosody. Key attributes of prosody include the relative pitch, duration, and intensity of the speech signal. Together, these features encode stress, intonation, and rhythm, all of which impact emotion perception. While we have identified general patterns to relate prosody to emotion, machine classification and synthesis of emotional speech remain unreliable.
This project tackles the problem of multi-speaker emotion conversion, which refers to modifying the perceived affect of a speech utterance without changing its linguistic content or speaker identity. To start, we have curated the VESUS dataset, which represents one of the largest collections of parallel emotional speech utterances. From here, we have introduced a new paradigm for emotion conversion that blends deformable curve registration of the prosodic features with a novel variational cycle GAN architecture that aligns the distribution of prosodic embeddings across classes. We are now working on methods for open-loop duration modification that leverages a learned attention mechanism.
Beyond advancing speech technology, our framework has the unique potential to improve human-human interactions by modifying natural speech. Consider autism, which is characterized by a blunted ability to recognize and respond to emotional cues. Suppose we could artificially amplify spoken emotional cues to the point at which an individual with autism can accurately perceive them. Over time, it may be possible to retrain the brain of autistic patient to use the appropriate neural pathways.
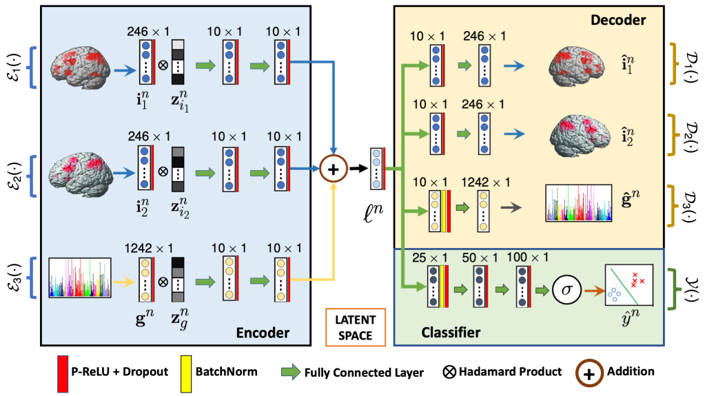
SELECTED PUBLICATIONS
Multispeaker Emotion Conversion via a Chained Encoder-Decoder-Predictor Network and Latent Variable Regularization.
R. Shankar, H.-W. Hsieh, N. Charon, A. Venkataraman.
In Proc. Interspeech: Conference of the International Speech Communication Association, 3391-3395, 2020.
Non-parallel Emotion Conversion using a Pair Discrimination Deep-Generative Hybrid Model.
R. Shankar, J. Sager, A. Venkataraman.
In Proc. Interspeech: Conf of the International Speech Communication Association, 3396-3400, 2020.
VESUS: A Crowd-Annotated Database to Study Emotion Production and Perception in Spoken English.
J. Sager, J. Reinhold, R. Shankar, A. Venkataraman.
In Proc. Interspeech: Conf of the International Speech Communication Association, 316-320, 2019. Selected for an Oral Presentation (<20% of Papers)
A Multi-Speaker Emotion Morphing Model Using Highway Networks and Maximum Likelihood Objective.
R. Shankar, J. Sager, A. Venkataraman.
In Proc. Interspeech: Conference of the International Speech Communication Association, 2848-2852, 2019. Selected for an Oral Presentation (<20% of Papers)
Forecasting Postoperative Kidney Failure
Acute kidney injury (AKI) is one of the most frequent post-surgicalcardiac surgery complications, with estimates ranging from 20-50% depending on the procedure. AKI is a serious morbidity associated with longer hospital stays, increased mortality, and greater risk of developing long-term chronic kidney injury. Hence, there is a clear need for perioperative physicians to understand the baseline and ongoing risk for AKI. Such information could help guide multiple aspects of the surgical planning, monitoring, and execution.
This proposal will develop automated methods to continuously forecast the risk for AKI based on preoperative clinical history and real-time intraoperative physiological data. To this end, we have formulated a novel deep learning architecture for AKI prediction and evaluated it on a large clinical dataset of >3,000 surgical patients.
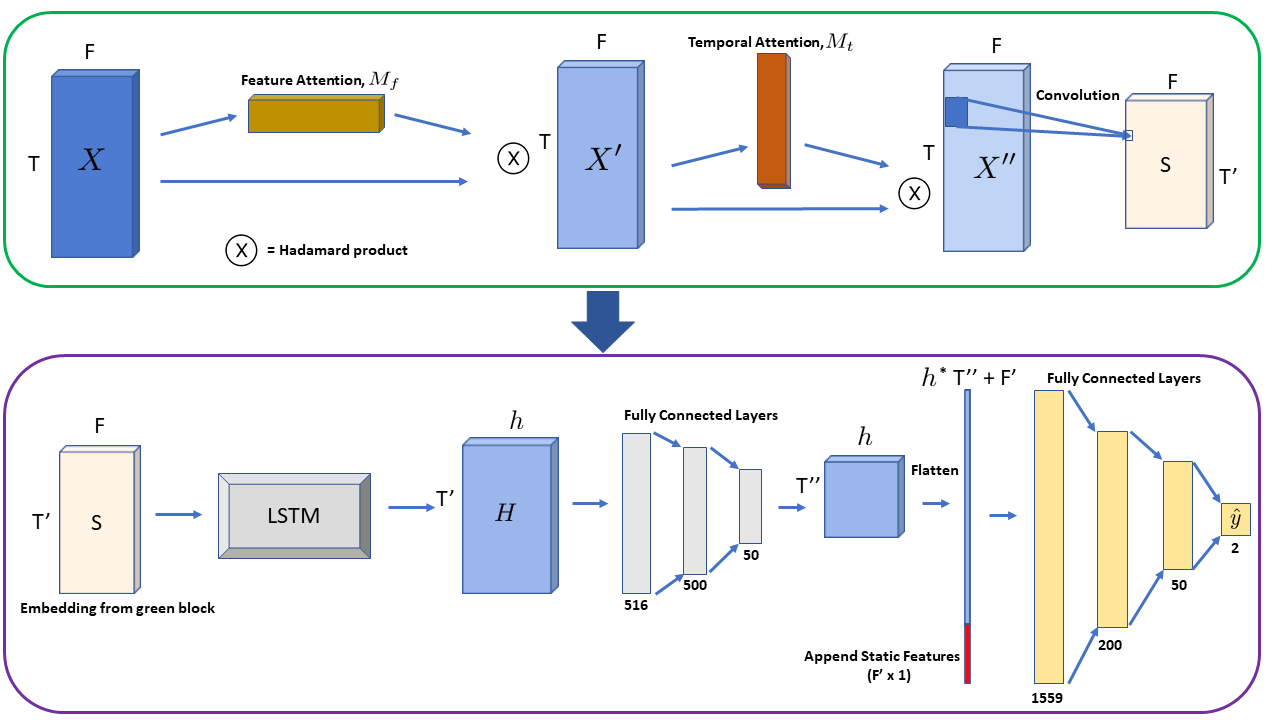
SELECTED PUBLICATIONS
Predicting Acute Kidney Injury via Interpretable Ensemble Learning and Attention Weighted Convoutional–Recurrent Neural Networks.
Y. Peng, N.S. D’Souza, B. Bush, C. Brown, A. Venkataraman.
In Proc. Conference on Information Sciences and Systems (CISS), pp. 1-6, 2021.
Examination of the Association Between Arterial Blood Pressure Below the Lower Limit of Autoregulation and Acute Kidney Injury After Cardiac Surgery.
R. Nandkarni.
MSE Thesis. Johns Hopkins University, Baltimore MD, 2019.
FUNDING
Malone Center Seed Grant (Joint PI: Venkataraman/Bush/Brown)
A Deep Learning Approach to Continuously Forecast Postoperative Kidney Failure During Cardiac Surgery
Project Dates: 01/01/20 – 12/31/21
Total Funding Amount: $50,000
Network Modeling of Enteric Nervous System
The Enteric Nervous System (ENS) is a collection of neurons and glial cells that reside within the wall of the gastrointestinal tract, which regulates intestinal motility. Structural aberrations are associated with intestinal dysmotility in acute and chronic gut diseases, and in myriad metabolic and neuro-degenerative disorders. Hence, our goal is to leverage tools in computer vision and machine learning to model the network structure and organization of the ENS.
Our approach combines the use of unsupervised algorithms and supervised, deep learning algorithms for segmenting composite images of immuno-stained tissue. Current work focuses on creating an image processing pipeline for neuron clustering and enumeration, and network extraction to form a connectome.
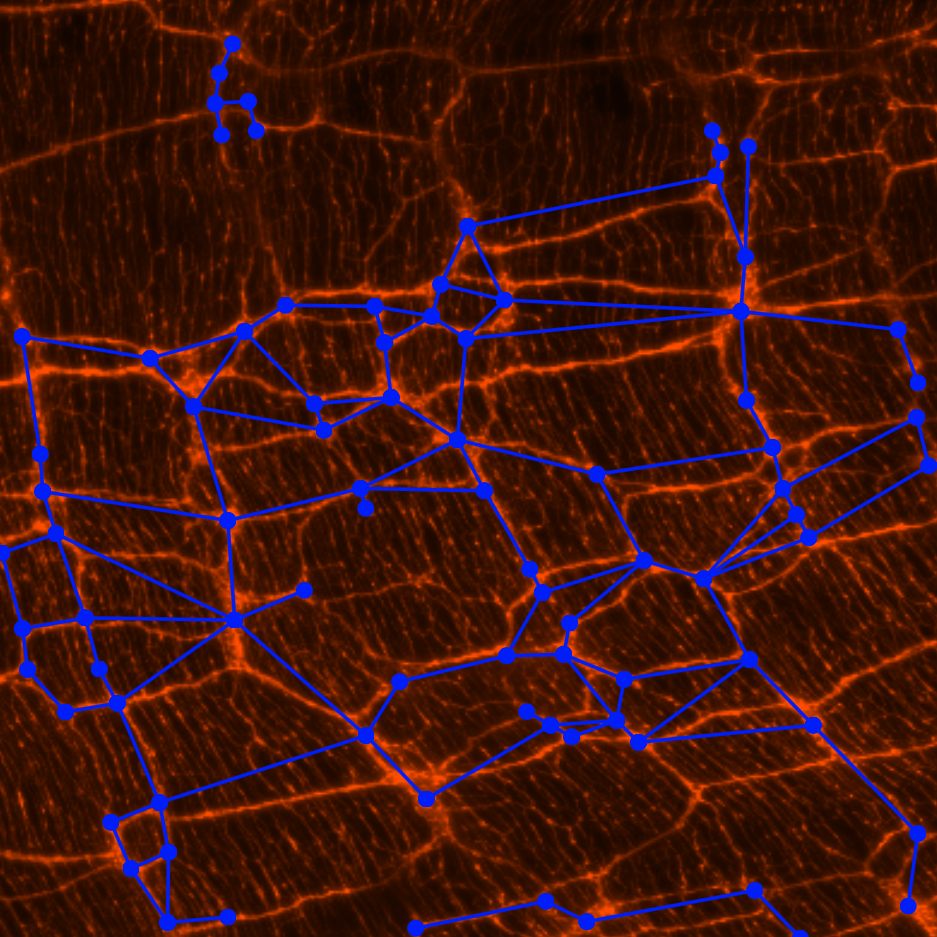
SELECTED PUBLICATIONS
A Statistical Map of the Adult Murine Ilieal Enteric Nervous System using an AI-Driven COUNTEN Algorithm.
Y. Kobayashi*, A. Bukowski*, S. Das*, N. Wagle, S. Bakshi, M. Saha, J. Kaltschmidt+, A. Venkataraman+, S. Kulkarni+.
eNeuro, In Press, 2021.
* Joint first authorship +Joint senior authorship
FUNDING